Active learning and weak supervision in NLP projects
Talk presentation
Successful artificial intelligence solutions always require a massive amount of high-quality labeled data. In most cases, we don’t have a large and qualitative labeled set together. Weak supervision and active learning tools may help you optimize the labeling process and address the shortage of data labels.
First, we will review how active learning can significantly reduce the amount of labeled data for training with classic approaches. We will show how active learning methods can be customized for a specific (NLP) task by using text embedding.
With weak supervision, we will see how using simple rules gets a big train dataset automatically and high model performance without manual labeling at all.
In the end, we will combine active learning and weak supervision by taking advantage of both techniques and achieving the best metrics.
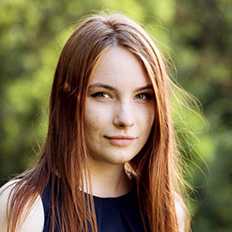
- The data scientist in the machine learning team at Wix
- Specializing in NLP solutions and has experience in building NLP-based recommendation scalable systems and end-to-end ML applications
- She is interested in insolving the low-resource problems and non-supervised techniques